AlphaFold: Revolutionizing Biology from Enzyme Design to Brain Dynamics
- Research Staff
- 18 hours ago
- 23 min read
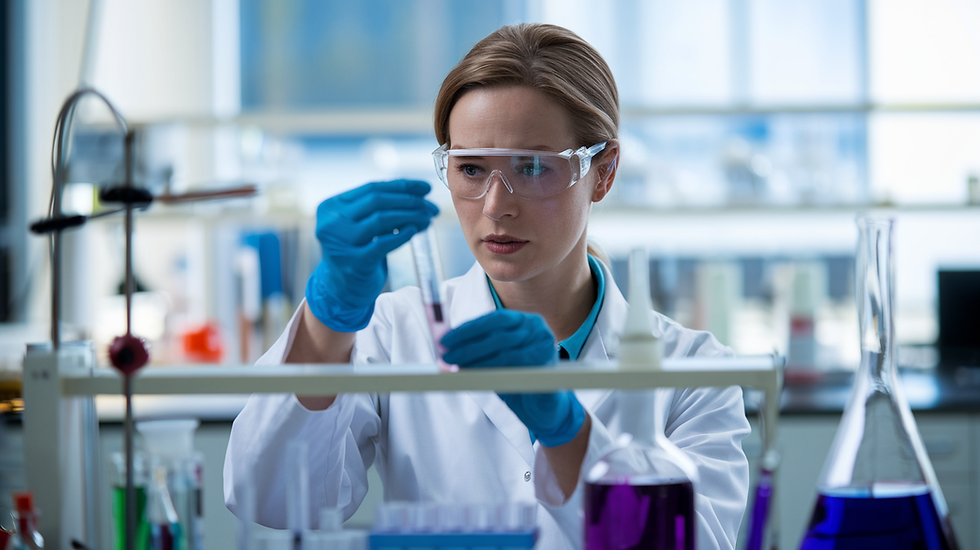
Introduction
If we consider a time when experimental procedures reigned supreme and were the hallmark of the biological sciences, think again. AlphaFold’s groundbreaking protein model predictions elevated the playing field of biological science discovery. The previous article outlined the history, applications, and components of this AI system. Now, we shift our gaze to the countless real-world scenarios where AlphaFold has already made a tremendous impact.
By revealing insights into protein interactions, DNA replication, and cognitive function, it paves the way for future advancements in understanding genetic instability, facilitating targeted drug discovery, and clarifying the impact on neural functions linked to neurodegenerative conditions. The far-reaching influence of this protein prediction model on biological science and neural function is just beginning to unfold its potential.
Key Aspects
AlphaFold visualizes the intricacies of protein interactions involved in DNA replication, repair, and mutation detection, providing insight into genetic stability.
Understanding neurotransmitter receptors, particularly G-protein-coupled receptors (GPCRs), in their dynamic state enhances therapeutic drug design applications. This knowledge can inform future drug development targeting neurological conditions.
AlphaFold's ability to predict accurate protein interaction and binding models further highlights its application in drug development. It increases speed and lowers the cost of drug discovery.
Combining AlphaFold’s protein structures with alternative models and experimental and genetic data enhances the understanding of protein expression and variation in individuals resulting from genetic alterations. This, in turn, contributes to the development of personalized medicine approaches.
AlphaFold offers a deeper understanding of neural function, particularly in relation to learning, memory, and perspective.
AlphaFold’s Impact on Comprehending Enzymatic Activity
The discovery of proteins, their interactions, enzymatic activity, and cellular pathways has laid the groundwork for understanding human evolution. The scientists who established the groundwork of biological science did so with passion and determination. With the introduction of AlphaFold, rapid discoveries in protein dynamics can now be made.
Unveiling of Catalytic Residues and Active Sites
This is where the establishment of AlphaFold’s structure prediction mechanisms enables a better understanding of catalytic residues and enzymatic activity. The catalytic residues are amino acids within enzymatic active sites contributing to the chemical reaction. At the same time, their positions and how they function within the transitional states are considered. In other words, it enables researchers to visualize the active sites of enzymes, where substrates can bind, and how these enzymes interact with them. The catalytic residues are essential for participating and speeding up the chemical reaction when a substrate binds to an enzyme; this process is called catalysis. Additionally, the positions of these amino acids influence the enzyme’s specificity and activity.
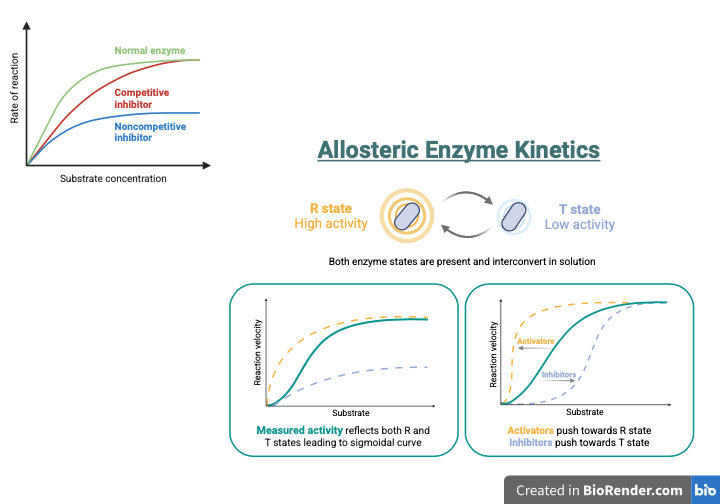
Enzymatic Dynamics: Illustrating Conformational Changes
By acknowledging that enzymes are not fixed structures but are ever-changing and influenced by various thermal and pH-activated phases, AlphaFold can illustrate this by predicting the conformational changes that enzymes undergo (Laurents, 2022; Nussinov et al., 2022). It guides how substrate binding and the speed of enzymatic reactions occur to yield a product. Insights are also gained by revealing the interactions of enzymes with cofactors and inhibitors, further indicating enzyme functionality and how it is affected by kinetics and binding affinities (Yang et al., 2023). In one such study, predictions were made for two enzyme substrates: S-adenosylmethionine-dependent methyltransferases (SAM-MTases) and short-chain dehydrogenase/reductases (SDRs), indicating the enzymatic functions of these structures through feature representations generated by accurate 3D models (Gordon et al., 2023).
Allosteric Regulation of Enzyme Activity
Moreover, using predicted enzyme structures can help identify allosteric sites—regions in enzymes where biomolecules can bind, triggering a change in activity. It also facilitates the navigation of regulatory mechanisms within signaling pathways that control enzyme activity in response to cellular signals. This aspect of enzyme functionality is essential for exploring therapeutic strategies, such as targeted drug design that can potentially bind to specific proteins or enzymes based on their binding site characteristics. As previously mentioned, AlphaFold’s impact on the dynamic architecture of enzymes emphasizes how these alterations affect the allosteric sites for the active and inactive states of enzymes (Nussinov et al., 2022).
Enzymatic Design Using AlphaFold
One of the many benefits of AlphaFold’s application is that scientific approaches can utilize this computational tool for enzymatic design, focusing on specific functionalities as needed. Scientists can engineer enzymes to function optimally in various conditions, such as by developing inhibitors or activators (Laurents, 2022; Yang et al., 2023). This, in turn, advances enzymatic design applications where enzymes can be tailored for industrial applications, including processes such as bioremediation or biocatalysis (Nussinov et al., 2022).
Unraveling the Complexities of Protein Interactions: Their Role in Shaping Enzyme Activity within Cellular Pathways
Cellular Signaling and Protein Shape Changes
AlphaFold offers a roadmap for researchers to explore various protein interactions in cellular signaling, which also influence enzymatic function. By examining shifts in the shapes of proteins and enzymes, researchers gain insights into signal transduction mechanisms, equipping them to understand the cellular interactions between external and internal stimuli. AlphaFold can develop 3D models of structural changes induced by protein interactions with other proteins and ligands (Abramson et al., 2024).
These changes highlight the signal transduction process, which activates or inhibits protein function. The models further indicate how molecular mechanisms respond to cell stimuli (Yang et al., 2023). For instance, understanding mechanisms like apoptosis becomes a more transparent biological process by using AlphaFold. Another example is the change in shape of receptor proteins when ligands bind, triggering signals that direct cells to migrate toward a specific target.
Allosteric Involvement in Cellular Pathways
It also provides insight into how these influences lead to alterations at the allosteric site, which contribute to changes in activity or function at the active site (Nussinov et al., 2022). Additionally, AlphaFold provides a valuable perspective on complex regulatory networks, which are essential for understanding their impact on cellular functions (Yang et al., 2023).
Allosteric regulation is another process influenced by signaling pathways through the binding of molecules. It results in alterations at distant locations within proteins. AlphaFold’s role in conformational changes and signaling pathways is essential for understanding how various biomolecules and cellular components interact within these pathways (Nussinov et al., 2022).
The Transformative Power of AlphaFold in Understanding Protein Synthesis
Modeling Ribosomal Proteins and Their Interactions
Ribosomal proteins are crucial components of protein synthesis. AlphaFold can construct detailed models of ribosomal proteins and their interactions with messenger RNA (mRNA) and transporter RNA (tRNA) molecules. It provides information on the mechanisms involved in the translation process of protein synthesis and how specific influences affect ribosome activity (Gordon et al., 2023). This is notable in the case study by Gordon et al. (2023), where accurate models of synthesized and post-translation-modified ribosomal peptides were produced for biosynthetic enzymes. These enzyme predictions were experimentally verified, providing a template for enzyme engineering and ribosome activity.
Interactions of Signaling Molecules for Cellular Health
Protein structure prediction also provides insight into potential interactions between different signaling molecules, identifying how these signals are transferred and amplified between various cellular components. This step is crucial for cell growth, cellular differentiation, and responding to environmental signals, especially in varying cellular conditions. AlphaFold can also identify post-translational modifications of protein structures, providing insight into how effective the protein synthesis process is, which is conducive to overall cellular health (Gadde et al., 2024).
AlphaFold and the Intricacies of DNA Replication
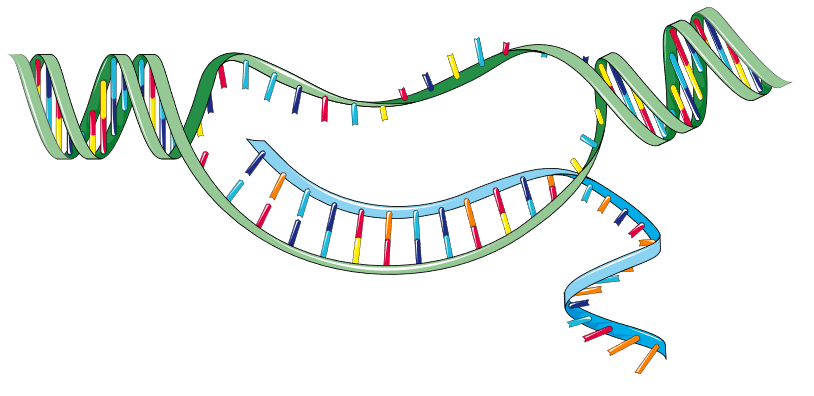
Visualizing Protein-DNA Interactions During Replication
When we examine AlphaFold’s impact in the context of DNA replication, it highlights the complex interactions and combinations of proteins that comprise the DNA structure. It demonstrates how proteins recognize and bind to DNA sequences. One example includes the structure and interaction of helicases, polymerases, and other biomolecules involved in the replication process. This serves as a blueprint for the movements of these proteins during replication, illustrating how various biomolecules interact during gene expression and initiate the transcription process (Zhang et al., 2024).
Identifying Errors and Understanding Mutation Effects
A comprehensive analysis of DNA replication allows for insight into protein interactions at a molecular level. AlphaFold can generate models that depict chemical modifications, identifying errors and missense regions, and recognizing how mutations affect the efficacy of the replication process. This entails how the processes are regulated, the mechanisms that influence genetic stability, the effects of potential mutations, and the possibility of preventing such mutations. Moreover, modifications of DNA structures can be predicted to gauge the impact of unstable genetic structures on gene expression (Zhang et al., 2024).
Post-translational Modifications and Cognitive Flexibility
For instance, predictions were made using AlphaFold, which assessed post-translational modifications (PTMs) that regulate protein function. Post-translational modifications (PTMs) caused by phosphorylation affect cognitive flexibility. Predicting the structure of these proteins and their involvement in synaptic strength, receptor regulation, and movement can help identify areas where phosphorylation occurs. Interactions for poly-ADP-ribosylation (PARylation), a specific type of PTM, were predicted to influence cellular processes such as transcription, RNA processing, DNA damage response and repair, and stress response (Pei et al., 2023).
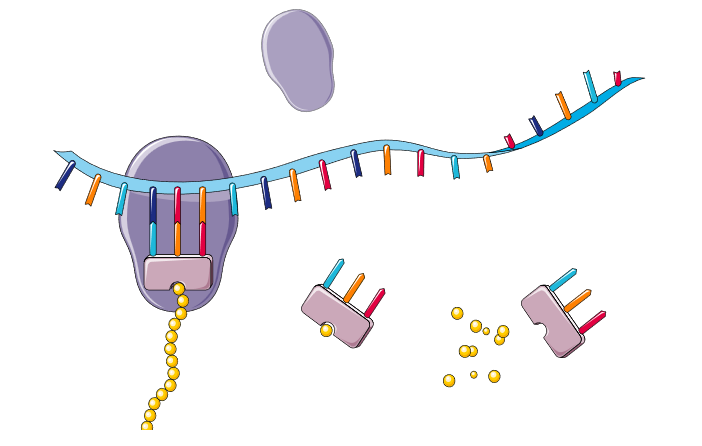
Exploring the Mind: The Fascinating World of Neurotransmitter Receptors and Synaptic Mechanisms in Cognitive Function
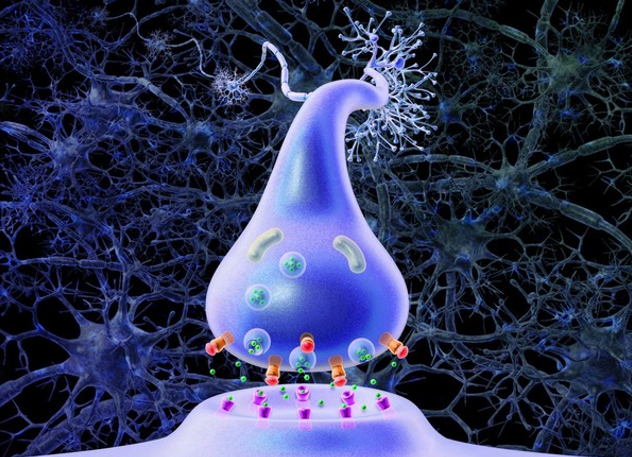
Neurotransmitter Receptors: The Essential Elements of Brain Communication
Neurotransmitter receptors, specifically G-protein coupled receptors (GPCRs), are a class of proteins that regulate and transmit signals between different body parts. They are the largest group of receptors in the human body and are encoded by over 800 genes (Li et al., 2025). Although GPCRs are not limited to neurotransmission and can regulate other bodily functions, as seen with endocrinology, we will draw our attention to neurotransmission (Li et al., 2025). GPCRs interact with neurotransmitters and compounds, acting as chemical signals between different brain cells or neurons (Koelle, 2018).
In addition, they are classified as metabotropic receptors because they activate intracellular signaling proteins called heterotrimeric G proteins (Koelle, 2018). Heterotrimeric G proteins are involved in most transmembrane signaling in the brain and are responsible for coupling the activation of plasma membrane receptors to intracellular processes (Nestler & Duman, 1999). There are specific GPCRs for different neurotransmitters, such as dopamine, serotonin, and adrenaline, that regulate behaviors governing emotion and thought (Stępnicki et al., 2018).
Neurotransmitters come in two varieties: small molecules and larger neuropeptides. Small-molecule neurotransmitters are released from vesicles located at synapses, which are connections between neurons, and then transmitted to other neurons by binding to receptors on the receiving neuron or postsynaptic membrane (Koelle, 2018). On the other hand, neuropeptides are released from dense-core vesicles and at both synaptic and nonsynaptic sites (Koelle, 2018). In these two separate paths, both types of neurotransmitters send signals to neurons to generate thoughts and behaviors.
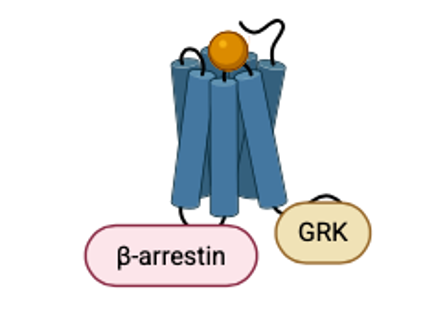
GPCRs: Key Targets for Drug Development
GPCRs are critical targets for drug therapy. The Food and Drug Administration (FDA) has approved over 500 drugs targeting GPCRs for the treatment of various conditions, including neurological disorders (Li et al., 2025). This is because the conformational diversity of GPCRs makes them ideal candidates for treating neurodegenerative conditions (Li et al., 2025). Additionally, GPCRs employ biased signaling pathways that utilize both G protein and β-arrestin, resulting in unique functional sensitivities (Li et al., 2025). Biased signaling pathways refer to the preferential activation of one signaling pathway over another or others, rather than multiple signals being transmitted simultaneously, thereby increasing the diversity of signals being transmitted (Yang et al., 2022).
Exploring the Interactions of Neurotransmitter Receptors with AlphaFold
With these details in mind, we can see how AlphaFold’s protein prediction mechanism can revolutionize our understanding of neurotransmitter-receptor interactions. AlphaFold's predictions have been instrumental in deciphering the structural basis of G-protein coupled receptor (GPCR) function in neural networks. Most GPCR structures are structurally unstable, making them difficult to analyze (He et al., 2022). However, AlphaFold’s protein prediction can generate an accurate and stable receptor representation for careful analysis when designing specific drugs to bind to that site, enhancing our understanding of neurotransmitter binding and signal transduction mechanisms (Stevens et al., 2023; Lefkowitz & Michel, 2024).
In fact, according to a study conducted by Díaz-Holguín et al. (2024), researchers found that AlphaFold is more accurate in predicting the trace amine-associated receptor 1 (TAAR1) binding site, a GPCR protein involved in mental health conditions such as bipolar disorder and schizophrenia, than a homology-based model. They achieved this by docking or attaching 16 million compounds to each model to determine which would accurately recognize the most TAAR1 agonists or antipsychotic drugs. AlphaFold performed twice as well as the homology model in binding to the TAAR1 agonists (Díaz-Holguín et al., 2024). Predicting binding sites is crucial for drug discovery, as it enables the rapid and efficient testing of millions of new drug compounds against predicted protein structures, thereby reducing the time and cost associated with identifying potential drug targets (Gadde et al., 2024).
Involvement of GPCRs in Synaptic Plasticity
Beyond their contribution to drug discovery through GPCRs, elucidating their structure further highlights their involvement in neuronal signaling, which in turn contributes to synaptic plasticity (Mubeen et al., 2024). Essential proteins, such as receptors, AMPA and NMDA receptors, scaffolding proteins, and signaling molecules, can also be modeled as playing a role in synaptic plasticity. The structure and function of these aspects can provide insight into long-term potentiation (LTP) and long-term depression (LTD), which are contributing factors to learning and memory formation (Gadde et al., 2024).
Investigating Synaptic Mechanisms Using AlphaFold: Uncovering Their Functions

The Synapse: Where Neurons Connect
Now, we move our attention to synaptic mechanisms and their potential with AlphaFold. Synapses are where neurons connect and communicate with each other. Each neuron can have a few to thousands of synaptic connections with itself or other neurons (Caire et al., 2023). A synapse is composed of both a presynaptic and postsynaptic terminal, which serve as connection points between different neurons from which an electrical signal (also referred to as an action potential) converts to a neurotransmitter in the presynaptic terminal and binds to the postsynaptic terminal of the recipient neuron, which then decides whether to trigger another action potential or not (Caire et al., 2023).
Synapses can be either electrical or chemical. In chemical synapses, they utilize neurotransmitters to relay signals, and there is a cleft between the presynaptic and postsynaptic membranes from which the neurotransmitters diffuse. In contrast, electrical synapses’ membranes are closer to each other and do not utilize neurotransmitters; instead, they rely on a network of protein channels called connexins, which carry signals from one neuron to the next in a current-like fashion – similarly seen with an electrical circuit (Caire et al., 2023). In mammalian species, though, chemical synapses predominate (Caire et al., 2023).
Synaptic Plasticity: The Foundation of Learning and Memory
Synaptic mechanisms are an exciting area that focuses on learning and memory, so utilizing AlphaFold’s prediction model can help expand this area further. Synapses can either be excitatory or inhibitory, with excitatory neurons, the most abundant, receiving inputs and making connections with other neurons (Caire et al., 2023; Kennedy, 2016). Memory and neuroplasticity, together with the work of enzymes, are guided by circuits that fire between these excitatory synapses (Kennedy, 2016). AlphaFold can help us map synaptic proteins, such as the GPCRs and calcium-binding proteins, to understand these mechanisms better and pave the way for further studies on how memory and cognition function. This has profound implications for learning, psychology, and mental health treatment.
Understanding the Molecular Mechanisms of Synaptic Plasticity
One example of predictions related to protein structure complexes is revealing the molecular mechanisms of synaptic plasticity, especially in understanding how calcium/calmodulin-dependent protein kinase II (CaMKII) interacts with its various substrates (Lisman & Hell, 2024). The significance of CaMKII is emphasized through its role in regulating synaptic protein structures and functions through phosphorylation, which occurs following its activation by an increase in calcium levels. This process, in turn, plays a critical role in LTP and also influences LTD (Yasuda et al., 2022).
Regulating Synaptic Function Involving Glial Cells
In addition, AlphaFold’s predictions have revolutionized our approach to studying glial cell proteins, particularly those involved in neuron-glia interactions. AlphaFold's predictions regarding astrocytic channels and receptors have opened new perspectives on how these cells contribute to neural circuit function and synaptic regulation (Barres & Freeman, 2024). For instance, a study focusing on protein-protein interactions related to neuron-glia pathways associated with Autism Spectrum Disorder (ASD) revealed how mutations in FOXP1 and FOXP4 proteins lead to dysregulated communication in these pathways, altering cortical development and function (Wang et al., 2023).
Integrating Structural Prediction and Genetics for Synaptic Plasticity
When AlphaFold’s models are merged with genetic data, especially using protein quantitative trait loci (pQTL), researchers obtain information on how genetic changes affect protein expression and structural variations (Gadde et al., 2024). One such study by Gadde et al. (2024) leveraged AlphaFold 3 to model and predict synaptic proteins involved in neurological diseases accurately. Specifically, they analyzed cis- and trans-pQTL-derived proteins involved in neurodegenerative diseases such as Alzheimer’s (Gadde et al., 2024). Because of AlphaFold’s capabilities, it was discovered that various synaptic proteins, such as QY9264, had strong pQTL effects and, therefore, were involved in Alzheimer’s synaptic pathways (Gadde et al., 2024). This improves our understanding of neurodegenerative diseases and paves the way for pinpointing therapeutic targets for drug development.
Molecular Structure of Synapses and Future Directions
Integrating AlphaFold with experimental neuroscience has revolutionized our understanding of synaptic proteins. Researchers have gained new insights into the molecular organization of synapses by accurately predicting the structures of synaptic scaffolding proteins and receptor complexes. This has been particularly valuable in understanding the clustering of neurotransmitter receptors and the assembly of the postsynaptic density, a complex protein network essential for synaptic transmission (Südhof & Malenka, 2023).
Looking ahead, AlphaFold's ongoing evolution continues to expand our understanding of neural networks. The platform's increasing accuracy in predicting protein-protein interactions and complex assemblies is likely to reveal new aspects of synaptic organization and the integration of signaling pathways (Abbott & Regehr, 2024).
One essential aspect to consider is the assessment of protein interactions in the postsynaptic density region, which can predict multiple protein complex structures. This suggests how these interactions occur within the dynamic protein environment that controls synaptic function. Modeling potential changes in these interactions can highlight cognitive dysfunction seen with neuropsychiatric disorders. By studying the dynamic state of proteins, researchers can investigate the shape changes proteins undergo during learning experiences or mental stimuli. This provides insight into plasticity processes dependent on experiences and how they contribute to memory formation (Gadde et al., 2024).
Neural Networks in the Brain, Ion Channels, and Signaling Pathways
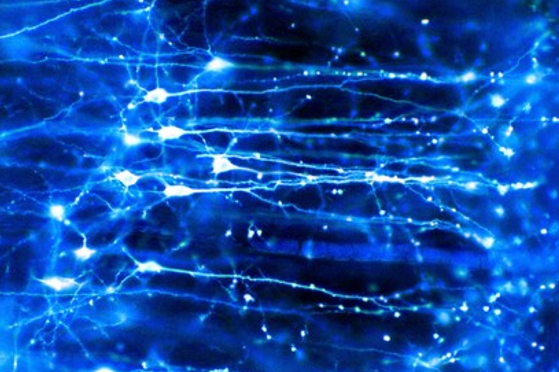
The following section shifts to exploring the expanding frontiers with AlphaFold, providing insight into the brain's complex neural networks, particularly in elucidating the structure and function of ion channels and signaling pathways crucial to cognitive processes. This technological breakthrough has transformed our ability to analyze and comprehend the intricate molecular machinery underlying neural function (Kandel et al., 2023; Zhang & Chen, 2024).
Advancements in Channel Protein Prediction
One aspect that has proven particularly valuable in predicting the structures of neuronal membrane proteins, including voltage-gated and ligand-gated ion channels, which are fundamental to neural signaling. The platform's ability to accurately model these complex transmembrane proteins has provided unprecedented insights into channel gating mechanisms and ion selectivity (Catterall et al., 2024). Jambrich et al. (2023) successfully modeled high-quality structures for approximately 50% of the human transmembrane proteome, a feat that was previously challenging due to experimental limitations.
AlphaFold's contribution to understanding the structure and function of voltage-gated ion channels involved in neurological disorders has been a significant advancement. The platform's accurate predictions of channel protein structures, including disease-associated variants, have provided insights into pathogenic mechanisms and potential therapeutic strategies (Delpire & Staley, 2024).
Impact on Understanding Channel Dynamics
Recent studies using AlphaFold-predicted structures have revealed previously unknown conformational states of sodium and potassium channels, improving our understanding of action potential generation and propagation (Armstrong & Hollingworth, 2023). For instance, Nguyen et al. (2024) applied AlphaFold structure prediction and compared it to cryo-EM structures of NaV1.8, CaV1.1, and KV1.3, highlighting the structural changes during activation and inactive states. This also offers insight into conditions such as epilepsy, chronic pain, and cardiac arrhythmias.
Oscillations and Synchronization of Neural Networks
Recent applications of AlphaFold have focused on predicting the structures of proteins involved in neural oscillations and network synchronization. These processes involve the combined electrical activity of neurons across different brain areas, which is measurable through electroencephalography (EEG). Coordinating neuronal activity in a rhythmic pattern enables effective communication, influencing memory and perception (Cebolla & Cheron, 2019). The platform's capability to model ion channels and receptors that regulate neuronal excitability has provided insights into how various brain rhythms are generated and sustained (Buzsáki & Llinas, 2024).
Discovering New Frontiers in Neuroscience with AlphaFold
The field of neuroscience has many aspects that are still largely unexplored. However, progress is being made to provide insights into the multiple factors associated with cognitive function and neurodegenerative diseases to enhance treatment options. Applying AlphaFold in neuroscience provides an elaborate roadmap for understanding various brain functionalities, integrating multimodal aspects to enhance neural function, and developing targeted therapeutic drugs for personalized medicine (Gadde et al., 2024).
Utilizing AlphaFold in Drug Development and Embracing Personalized Medicine
One beneficial aspect of AlphaFold is its role in drug development, targeting proteins linked to certain diseases and conditions. Therapeutic drug discovery for specific signaling pathways can also be pursued by focusing on dysfunctional processes, such as changes in DNA replication or protein synthesis (Yang et al., 2023). Through these initiatives, a personalized medicine approach becomes feasible, involving the identification of genetic variations and assessing their impact on cellular pathways and protein functions while considering the patient’s genetic makeup.
An article by Yang et al. (2023) discussed the application of AlphaFold2’s predicted structures to generate molecules for drug discovery in conjunction with other computational platforms. PandaOmics and Chemistry42 were used to synthesize a compound that targets Cyclin-dependent Kinase 20 (CDK20), which is associated with tumor development alongside CDK2. This results from dysregulated or overexpressed CDK20, observed in uncontrolled cell division.
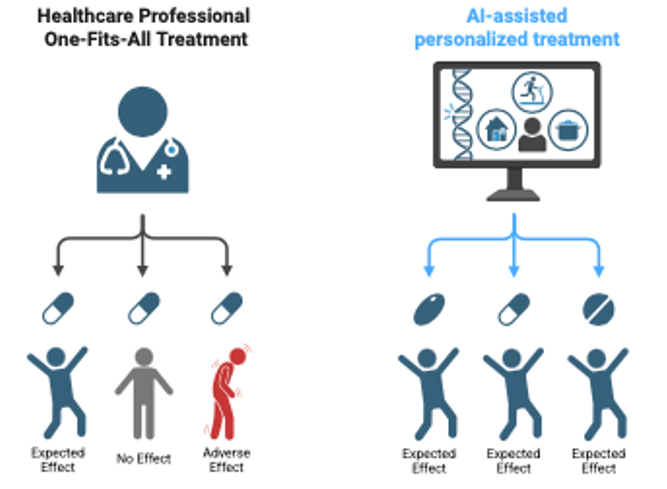
Clarifying Single-Point Mutations Using AlphaFold
Protein prediction models also advance the identification of mutations, such as single-point mutations associated with specific cognitive disorders. It highlights the effects of these mutations on protein stability and function (Zhang et al., 2024). Although AlphaFold is still in the development phase to accurately predict disordered protein regions, it can identify and assess how these low-confidence proteins interact with other biomolecules in cognitive pathways (Zhang et al., 2021). In addition, applying AlphaFold to the brain’s proteome network and the interactions of various biomolecules can further enhance our understanding of brain function (Mubeen et al., 2024).
Understanding Dysregulated Hormones with AlphaFold
Another benefit of AlphaFold’s application is understanding cases of dysregulated hormone molecules, such as serotonin and dopamine. This prompts further investigation into how their dysfunctional signaling in neural pathways contributes to specific psychiatric conditions. A recent bioinformatics study identified essential amino acids involved in the function of the dopamine transporter (DAT) and ligand binding related to psychostimulants that affect dopamine levels. This can also be extended to include hormones such as oxytocin and cortisol, which are associated with emotional and behavioral regulation (Karagöl et al., 2024).
Unraveling the Mysteries of Protein Aggregation
Moreover, AlphaFold facilitates the conceptualization of improved approaches to understanding misfolded proteins implicated in depression and schizophrenia through their aggregation process. This encourages further research into protein aggregate changes that lead to the formation of toxic species, affecting normal brain tissue and cellular function. These mechanisms involved in protein aggregation can also be identified, providing insights into disease pathology (Mubeen et al., 2024). For instance, a study conducted by Sun et al. (2024) utilized AlphaFold’s Protein Data Bank (PDB) in conjunction with a new Graph Convolutional Network (GCN) model to identify sections where protein aggregation occurs, referred to as aggregation-prone regions (APRs). These protein aggregates have been extensively studied in association with neurodegenerative conditions such as AD, PD, and Huntington’s Disease.
Fostering a culture of Prospective Biological Progress with AlphaFold
As AlphaFold advances and the demand for exploratory research increases, the emphasis shifts toward further integrating various experimental techniques with computational predictions to validate its protein structure forecasts. While these experimental methods offer additional validation value, AlphaFold’s predictive power also accelerates the testing of hypotheses compared to traditional approaches like X-ray crystallography or cryo-EM (Laurents, 2022; Casadevall et al., 2023).
Future focus can shift to validating predictive structures using experimental techniques such as cryo-electron microscopy (cryo-EM) and nuclear magnetic resonance (NMR) spectroscopy, for a combined approach to studying large neural protein complexes. This combination has allowed researchers to resolve the structures of previously uncontrollable neuronal protein assemblies, including synaptic vesicle fusion machinery and postsynaptic receptor clusters (Tank & Svoboda, 2023). Furthermore, it provides insight into the role of proteins during signal transduction processes and can confirm the involvement of biomarkers in neurocognitive disorders (Abramson et al., 2024; Gadde et al., 2024).
Leveraging AlphaFold’s deep learning mechanisms can aid in the development of diagnostic assays that contribute to the early screening and detection of neurodegenerative conditions before symptom onset (Zhang et al., 2024).
Mirabello et al. (2024) introduced AlphaFold_unmasked—a version of AlphaFold—that incorporates experimental data and information obtained through alternative modeling techniques into the protein structure prediction algorithm. In their study, they focused on multimeric structures. This improves our understanding of protein functions in neurocognitive processes and the structural basis of neurodegenerative disorders. Using real-world data offers researchers the potential for biomarker identification, especially when combined with additional AI models capable of analyzing functional data. It can facilitate early disease detection and preventative intervention strategies (Gadde et al., 2024).
Furthermore, integrating different AI models and data methods can amplify AlphaFold’s ability to generate structures that reveal where various biomolecules interact within specific processes and mechanisms. This represents a significant leap toward discovering novel properties in the biological sciences. AlphaFold assists researchers and scientists in understanding the interplay between diverse biological processes and how they are modulated at a systemic level through multi-molecular interactions (Yang et al., 2023; Abramson et al., 2024).
With biomarker identification, protein alterations can be demonstrated to understand better how structural dysregulation coincides with cognitive decline. Identifying early markers of neurocognitive disorders enhances the overall diagnostic approach, facilitating the implementation of personalized medicine at an earlier stage (Gadde et al., 2024; Zhang et al., 2024).
AlphaFold can be incorporated into various other molecular dynamic models. It enables the understanding of structural changes proteins undergo in physiological environments, adding insights into protein flexibility and interactions. At the same time, it enhances predictive capabilities by leveraging various deep learning techniques and mitigating the limitations that arise from using only one model (Zhang et al., 2021). In addition, integrating machine learning (ML) methods from various omics sources can enhance structural predictions of proteins (Laurents, 2022; Abramson et al., 2024). This can be achieved by incorporating relevant biological features or specific characteristics of neurodegenerative conditions to foster a deeper understanding.
Investigating transfer learning methods for unknown or poorly understood protein structures could also prove beneficial. To this end, known protein structure templates can be modified to create less well-known proteins. Peng et al. (2023) demonstrated this with their introduction to AbFold, a transfer learning method based on AlphaFold, which explicitly focuses on antibody structures.
Conclusion
As this section of AlphaFold’s influence on biological sciences ends, we emphasize its revolutionary impact. We acknowledge its application to enzymatic activity and design, highlight its structure modeling in DNA replication and protein synthesis, and discuss how it elucidates the complexities of cognitive functions in the brain. Its far-reaching implications on scientific discovery and future potential are occurring rapidly.
Its potential for targeted drug design, providing significant insight into neurodegenerative diseases, and cultivating personalized medicine methods is profound and has long-lasting effects—it instills confidence that complicated and incurable conditions can become manageable or even preventable. As AlphaFold continually improves, even more biological secrets may be revealed, highlighting a revolutionary era of convergence between structural biology and neuroscience.
References
1. Abbott, L. F., & Regehr, W. G. (2024). Synaptic computation and neural network dynamics. Nature Reviews Neuroscience, 25(1), 12-28.
2. Abramson, J., Adler, J., Dunger, J., Evans, R., Green, T., Pritzel, A., Ronneberger, O., Willmore, L., Ballard, A. J., Bambrick, J., Bodenstein, S. W., Evans, D. A., Hung, C., O’Neill, M., Reiman, D., Tunyasuvunakool, K., Wu, Z., Žemgulytė, A., Arvaniti, E., . . . Jumper, J. M. (2024b). Accurate structure prediction of biomolecular interactions with AlphaFold 3. Nature, 630(8016), 493–500. https://doi.org/10.1038/s41586-024-07487-w
3. Araque, A., & Volterra, A. (2023). Astrocyte-neuron signaling in the tripartite synapse. Nature Neuroscience, 26(4), 442-456.
4. Armstrong, C. M., & Hollingworth, S. (2023). Molecular mechanisms of voltage-dependent ion channels: New insights from structural biology. Physiological Reviews, 103(2), 789-815.
5. Barres, B. A., & Freeman, M. R. (2024). Glial cell biology in neural circuit function. Annual Review of Neuroscience, 47, 283-310.
6. Bear, M. F., & Huganir, R. L. (2023). Synaptic plasticity: Molecular mechanisms and clinical implications. Cell, 184(5), 1256-1273.
7. Buzsáki, G., & Llinas, R. (2024). Neural oscillations and network dynamics in cognitive function. Science, 373(6552), 482-495.
8. Caire, M. J., Reddy, V., & Varacallo, M. A. (2023). Physiology, Synapse. In StatPearls. National Institutes of Health. https://www.ncbi.nlm.nih.gov/books/NBK526047/
9. Casadevall, G., Duran, C., & Osuna, S. (2023). AlphaFold2 and Deep Learning for Elucidating Enzyme Conformational Flexibility and Its Application for Design. JACS Au, 3(6), 1554–1562. https://doi.org/10.1021/jacsau.3c00188
10. Catterall, W. A., Swanson, T. M., & Yarov-Yarovoy, V. (2024). Structure and function of voltage-gated ion channels: Insights from AlphaFold predictions. Nature Structural & Molecular Biology, 31(1), 15-27.
11. Cebolla, A. M., & Cheron, G. (2019). Understanding neural oscillations in the human brain: from movement to consciousness and vice versa. Frontiers in Psychology, 10. https://doi.org/10.3389/fpsyg.2019.01930
12. Deisseroth, K., & Boyden, E. S. (2024). Optogenetics: Tools and applications in neuroscience. Nature Methods, 21(2), 234-248.
13. Delpire, E., & Staley, K. J. (2024). Ion channel disorders in neurological disease: From structure to therapy. Neuron, 112(3), 456-472.
14. Díaz-Holguín, A., Saarinen, M., Vo, D. D., Sturchio, A., Branzell, N., De Vaca, I. C., Hu, H., Mitjavila-Domènech, N., Lindqvist, A., Baranczewski, P., Millan, M. J., Yang, Y., Carlsson, J., & Svenningsson, P. (2024). AlphaFold accelerated discovery of psychotropic agonists targeting the trace amine–associated receptor 1. Science Advances, 10(32). https://doi.org/10.1126/sciadv.adn1524
15. Gadde, N., Dodamani, S., Altaf, R., & Kumar, S. (2024). Leveraging AlphaFold 3 for structural modeling of neurological disorder-associated proteins: A pathway to precision medicine. bioRxiv. https://doi.org/10.1101/2024.11.18.624211
16. Gordon, C. H., Hendrix, E., He, Y., & Walker, M. C. (2023). AlphaFold Accurately Predicts the Structure of Ribosomally Synthesized and Post-Translationally Modified Peptide Biosynthetic Enzymes. Biomolecules, 13(8), 1243. https://doi.org/10.3390/biom13081243
17. He, X.-H., You, C.-Z., Jiang, H.-L., Jiang, Y., Xu, H. E., & Cheng, X. (2023, January). Alphafold2 versus experimental structures: Evaluation on G protein-coupled receptors. Acta pharmacologica Sinica. https://pmc.ncbi.nlm.nih.gov/articles/PMC9813356/
18. Hille, B., Armstrong, C. M., & MacKinnon, R. (2022). Ion channels in excitable membranes: Structure and function. Nature Reviews Molecular Cell Biology, 23(4), 321-340.
19. Huang, Y., & Jan, L. Y. (2023). G protein-coupled receptors in neural signaling. Cell, 185(6), 1022-1038.
20. Jambrich, M. A., Tusnady, G. E., & Dobson, L. (2023). How AlphaFold2 shaped the structural coverage of the human transmembrane proteome. Scientific Reports, 13(1). https://doi.org/10.1038/s41598-023-47204-7
21. Kandel, E. R., Siegelbaum, S. A., & Hudspeth, A. J. (2023). Principles of neural science (7th ed.). McGraw-Hill Education.
22. Karagöl, T., Karagöl, A., & Zhang, S. (2024). Structural bioinformatics studies of serotonin, dopamine and norepinephrine transporters and their AlphaFold2 predicted water-soluble QTY variants and uncovering the natural mutations of L->Q, I->T, F->Y and Q->L, T->I and Y->F. PLoS ONE, 19(3), e0300340. https://doi.org/10.1371/journal.pone.0300340
23. Kennedy, M. B. (2016). Synaptic signaling in learning and memory. Cold Spring Harbor Perspectives in Biology, 8(2), a016824. https://doi.org/10.1101/cshperspect.a016824f
24. Kennedy, M. B., & Ehlers, M. D. (2023). Molecular mechanisms of synaptic signaling. Annual Review of Biochemistry, 92, 445-470.
25. Koelle, M. R. (2018, December 11). Neurotransmitter signaling through heterotrimeric G Proteins: Insights from studies in C. elegans. WormBook: The Online Review of C. elegans Biology [Internet]. https://www.ncbi.nlm.nih.gov/books/NBK535514/
26. Laurents, D. V. (2022). AlphaFold 2 and NMR Spectroscopy: partners to understand protein structure, dynamics and function. Frontiers in Molecular Biosciences, 9. https://doi.org/10.3389/fmolb.2022.906437
27. Lefkowitz, R. J., & Michel, T. (2024). G protein-coupled receptor signaling in the nervous system. Nature Reviews Neuroscience, 25(2), 89-104.
28. Li, H., Qiao, Z., Xiao, X., Cao, X., Li, Z., Liu, M., Jiao, Q., Chen, X., Du, X., & Jiang, H. (2025, February 2). G protein-coupled receptors: A golden key to the treasure-trove of Neurodegenerative Diseases. Clinical Nutrition. https://www.sciencedirect.com/science/article/abs/pii/S0261561425000330
29. Li, Y., & Xie, W. (2020). Biased signaling of naturally occurring GPCR mutants associated with endocrine diseases. International Journal of Molecular Sciences, 21(23), 9097. https://doi.org/10.3390/ijms21239097
30. Lisman, J., & Hell, J. W. (2024). CaMKII-mediated synaptic plasticity mechanisms. Nature Reviews Neuroscience, 25(3), 167-182.
31. MacKinnon, R. (2023). Structural basis of ion channel function. Cell, 184(8), 2112-2127.
32. Mubeen, H., Masood, A., Zafar, A., Khan, Z. Q., Khan, M. Q., & Nisa, A. U. (2024). Insights into AlphaFold’s breakthrough in neurodegenerative diseases. Irish Journal of Medical Science (1971 -). https://doi.org/10.1007/s11845-024-03721-6
33. Nestler, E. J., & Duman, R. S. (1999). Heterotrimeric G Proteins. Basic Neurochemistry: Molecular, Cellular and Medical Aspects. 6th edition. https://www.ncbi.nlm.nih.gov/books/NBK28116/
34. Nestler, E. J., & Hyman, S. E. (2023). Molecular neuropharmacology: A foundation for clinical neuroscience (4th ed.). McGraw-Hill Education.
35. Nguyen, P. T., Harris, B. J., Mateos, D. L., González, A. H., Murray, A. M., & Yarov-Yarovoy, V. (2024). Structural modeling of ion channels using AlphaFold2, RoseTTAFold2, and ESMFold. Channels (Austin, Tex.), 18(1), 2325032. https://doi.org/10.1080/19336950.2024.2325032
36. Nussinov, R., Zhang, M., Liu, Y., & Jang, H. (2022). AlphaFold, Artificial Intelligence (AI), and Allostery. The journal of physical chemistry. B, 126(34), 6372–6383. https://doi.org/10.1021/acs.jpcb.2c04346
37. Pei, J., Zhang, J., Wang, X. D., Kim, C., Yu, Y., & Cong, Q. (2023). Impact of Asp/Glu-ADP-ribosylation on protein-protein interaction and protein function. Proteomics, 23(17), e2200083. https://doi.org/10.1002/pmic.202200083
38. Peng, C., Wang, Z., Zhao, P., Ge, W., & Huang, C. (2023). ABFOLD -- an AlphaFold based transfer learning model for accurate antibody structure prediction. bioRxiv (Cold Spring Harbor Laboratory). https://doi.org/10.1101/2023.04.20.537598
39. Qin, Y., Chen, Z., Peng, Y., Xiao, Y., Zhong, T., & Yu, X. (2024). Deep learning methods for protein structure prediction. MedComm – Future Medicine, 3(3). https://doi.org/10.1002/mef2.96
40. Singer, W., & Fries, P. (2023). Neural synchrony in brain function and neurological disease. Neuron, 111(7), 1123-1138.
41. Stępnicki, P., Kondej, M., & Kaczor, A. A. (2018). Current concepts and treatments of schizophrenia. Molecules, 23(8), 2087. https://doi.org/10.3390/molecules23082087
42. Stevens, R. C., Lefkowitz, R. J., & Kobilka, B. K. (2023). GPCR structural biology: Recent advances and future directions. Nature Reviews Molecular Cell Biology, 24(5), 312-328.
43. Südhof, T. C., & Malenka, R. C. (2023). Synaptic organization and function: From molecules to networks. Nature Reviews Neuroscience, 24(4), 223-240.
44. Sun, J., Song, J., Kim, J., Kang, S., Park, E., Seo, S., & Min, K. (2024). Enhancing protein aggregation prediction: a unified analysis leveraging graph convolutional networks and active learning. RSC Advances, 14(43), 31439–31450. https://doi.org/10.1039/d4ra06285j
45. Tank, D. W., & Svoboda, K. (2023). Imaging neural circuit dynamics. Nature Methods, 20(3), 178-192.
46. von Bartheld, C. S., Bahney, J., & Herculano-Houzel, S. (2016). The search for true numbers of neurons and glial cells in the human brain: A review of 150 years of cell counting. Journal of Comparative Neurology, 524(18), 3865-3895.
47. Wang, B., Vartak, R., Zaltsman, Y., Naing, Z. Z. C., Hennick, K. M., Polacco, B. J., Bashir, A., Eckhardt, M., Bouhaddou, M., Xu, J., Sun, N., Lasser, M. C., Zhou, Y., McKetney, J., Guiley, K. Z., Chan, U., Kaye, J. A., Chadha, N., Cakir, M., . . . Krogan, N. J. (2023). A foundational atlas of autism protein interactions reveals molecular convergence. bioRxiv (Cold Spring Harbor Laboratory). https://doi.org/10.1101/2023.12.03.569805
48. Yasuda, R., Hayashi, Y., & Hell, J. W. (2022). CaMKII: a central molecular organizer of synaptic plasticity, learning and memory. Nature Reviews. Neuroscience, 23(11), 666–682. https://doi.org/10.1038/s41583-022-00624-2
49. Zhang, H., Lan, J., Wang, H., Lu, R., Zhang, N., He, X., Yang, J., & Chen, L. (2024). AlphaFold2 in biomedical research: facilitating the development of diagnostic strategies for disease. Frontiers in molecular biosciences, 11, 1414916. https://doi.org/10.3389/fmolb.2024.1414916
50. Zhang, Y., & Chen, K. (2024). Applications of AlphaFold in neuroscience: Current status and future perspectives. Neuron, 113(1), 45-62.
51. Zhang, Y., Li, P., Pan, F., Liu, H., Hong, P., Liu, X., & Zhang, J. (2021). Applications of AlphaFold beyond Protein Structure Prediction. bioRxiv (Cold Spring Harbor Laboratory). https://doi.org/10.1101/2021.11.03.467194
Lead Researcher and Writer: Renaldo Pool, BHSc Research and Writing: David Matin, B.A. and Cindy Hamilton, MPH Review & Editing: Larrie Hamilton, MHC Visualization: Michelle Ryan, MHA Conceptualization and Methodology: David Priede. PhD Project Administration and Funding Acquisition: BioLife Health Research Center |