
This article explores the increasing ability of artificial intelligence models to surpass human doctors in accurately diagnosing complex and challenging medical cases.
Takeaways
AI models are demonstrating superior diagnostic accuracy in some complex medical scenarios.
This improved accuracy stems from AI's ability to process vast datasets and identify subtle patterns.
AI can assist, not replace, physicians as a powerful diagnostic tool.
The future of medical diagnosis likely involves a collaborative partnership between humans and AI.
As a health professional, I've always believed in the power of meticulous observation, thorough data analysis, and the human touch in patient care. However, the landscape of medicine is rapidly evolving. Recent advancements in artificial intelligence (AI) are forcing us to re-evaluate our assumptions about the limits of human diagnostic capability.
We're entering an era where AI models are not just assisting in diagnosis but, in some instances, demonstrably outperforming seasoned physicians, especially when confronted with the most intricate and puzzling medical cases. This article will explore this transformative shift, its implications, and what it means for the future of healthcare.
The Challenge of Complex Diagnoses
Medicine, at its core, is about pattern recognition. We, as clinicians, gather information – patient history, physical exam findings, lab results, imaging studies – and synthesize it to arrive at a diagnosis. But what happens when the patterns are subtle, contradictory, or buried within a mountain of data?
This is where complex cases emerge, often involving rare diseases, atypical presentations of common conditions, or multiple interacting illnesses. These cases can tax even the most experienced physicians, leading to diagnostic delays, misdiagnoses, and potentially adverse patient outcomes.
Real-World Example: Consider a patient, let's call him John, presenting with vague symptoms: fatigue, intermittent fevers, and subtle joint pain. These symptoms could point to a myriad of conditions, from a simple viral infection to something far more serious like lupus, Lyme disease, or even certain types of cancer. The diagnostic process might involve numerous tests, consultations with specialists, and a significant investment of time and resources, all while John experiences continued uncertainty and discomfort.
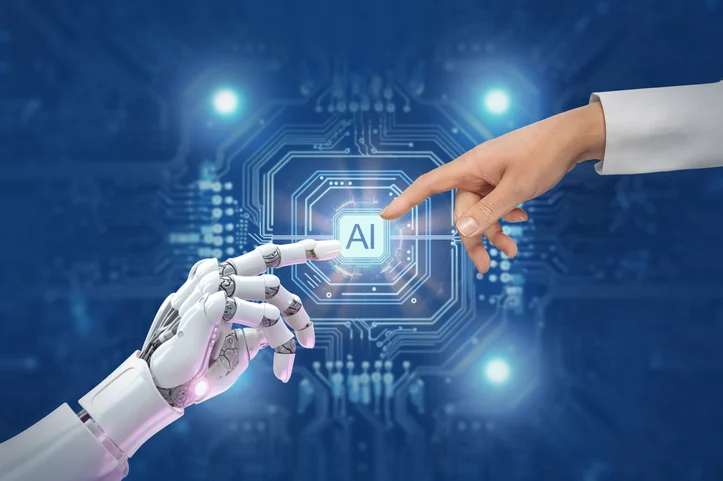
The AI Advantage: Data Processing and Pattern Recognition
This is precisely where AI is beginning to demonstrate its potential. AI models, particularly those based on deep learning, can be trained on vast datasets encompassing thousands, even millions, of patient cases, far exceeding the experience any single physician could accumulate in a lifetime. These models can identify subtle correlations and patterns within the data that might be invisible to the human eye.
For instance, AI algorithms can analyze medical images (X-rays, MRIs, CT scans) with remarkable speed and accuracy, often detecting subtle anomalies like early-stage tumors that human radiologists might miss. Studies have shown AI's ability to exceed human performance in specific image-based diagnostic tasks. Machine learning, a key component of AI used in healthcare, has significantly reshaped healthcare by enhancing medical diagnosis and treatment.
Beyond Imaging: AI in Diverse Medical Specialties
The application of AI extends far beyond radiology. AI is being used in:
Cardiology: AI algorithms can analyze electrocardiograms (ECGs) to detect subtle arrhythmias or predict the risk of heart disease with improved accuracy.
Neurology: AI can assist in diagnosing neurological disorders like Alzheimer's disease and multiple sclerosis by analyzing brain scans and patient data.
Pathology: AI can improve the accuracy and speed of disease detection.
Dermatology: AI models can analyze images of skin lesions to differentiate between benign and malignant growths with high accuracy.
Autism: AI showed an area under the curve (AUC) of 0.92 for identifying autism spectrum disorders.

The Human-AI Partnership: A Collaborative Future
It's essential to emphasize that AI is not intended to replace physicians. Rather, it's a powerful tool to augment our capabilities. The ideal future is one of collaboration, where AI handles the computationally intensive tasks of data analysis and pattern recognition, freeing up physicians to focus on the more nuanced aspects of patient care: communication, empathy, and complex decision-making that require clinical judgment and experience. AI can offer a "second opinion," prompting clinicians to consider possibilities they might have overlooked.
Real-World Example: Returning to John, our patient with ambiguous symptoms, an AI model could analyze his complete medical record, including lab results, genetic information, and even lifestyle data from wearable devices. The AI might flag a subtle pattern indicative of a rare autoimmune disorder, prompting the physician to order specific tests that confirm the diagnosis much earlier than would have been possible otherwise.
Ethical and Practical Considerations
The integration of AI into medical diagnosis is not without its challenges. Several key issues need careful consideration:
Data Bias: AI models are only as good as the data they are trained on. If the training data is biased (e.g., underrepresenting certain demographic groups), the AI's diagnostic accuracy may be compromised for those groups.
Transparency and Explainability: Many AI models, especially deep learning networks, operate as "black boxes," making it difficult to understand why they arrived at a particular diagnosis. This lack of transparency can erode trust and make it challenging for clinicians to validate the AI's recommendations.
Data Privacy and Security: Patient data is highly sensitive. Robust security measures are needed to protect this data from breaches and ensure compliance with privacy regulations.
Informed Consent: Patients should have the right to consent or to opt out.
Liability: Clear guidelines are needed to address liability in cases of misdiagnosis involving AI.
Summary
This article has explored the growing role of AI in medical diagnosis, particularly in complex cases where traditional methods may fall short. AI's ability to process vast datasets and identify subtle patterns offers significant advantages in diagnostic accuracy and speed. However, AI is best viewed as a collaborative tool, augmenting the skills of physicians rather than replacing them. The integration of AI into healthcare raises ethical and [8]practical considerations that must be addressed to ensure responsible and equitable implementation.
Final Thoughts
The rise of AI in medical diagnosis represents a paradigm shift in healthcare. While challenges remain, the potential benefits – improved accuracy, earlier diagnoses, and more personalized treatment – are too significant to ignore. As we move forward, a thoughtful, collaborative, and ethically grounded approach will be to unlock the full transformative power of AI in medicine.
Frequently Asked Questions:
Will AI replace my doctor? No, AI is designed to assist doctors, not replace them. It's a tool that enhances their diagnostic capabilities, allowing them to focus on patient interaction and complex decision-making.
Is AI always right? No, AI is not infallible. AI models can be affected by biases in the data they are trained on, and they may not always provide the correct diagnosis. It's essential to view AI as a second opinion, not the final word.
How does AI protect my privacy? AI systems in healthcare must adhere to strict data privacy regulations. Data encryption, anonymization, and secure storage are crucial to protecting patient information.
What if I don't want AI involved in my diagnosis? Patients have the right to be informed about the use of AI in their care and should have the option to decline its use.
How can I learn more about AI in healthcare? Reputable medical journals, professional organizations (like the AMA), and government health agencies (like the NIH) are good sources of information on AI in healthcare.
Sources:
Frontiers in Public Health. (2022, March 13). Ethical Challenges. Frontiers.
HITRUST Alliance. (2023, November 15). The Ethics of AI in Healthcare. HITRUST.
Kanda Software. (2024,January,15) How AI Assists in Diagnostics of Complex Clinical Cases. Kanda Software.
Labelbox. (2023, May 5). The Impact of AI in Healthcare.
Lifespan.io. (2024, December 5). AI Outperforms AI-Assisted Doctors in Diagnostic Reasoning.
LiveNOW from FOX. (2024, November 21). ChatGPT outperformed doctors in diagnostic accuracy, study reveals. LiveNOW from FOX
Medrxiv (2024, March 18). Key Points. Medrxiv.
News-Medical.net. (2024, November 6). AI outperforms doctors in diagnostics but falls short as a clinical assistant. News-Medical.net.
NIX United. (2023, October 13). AI Medical Diagnosis: Benefits, Challenges, and Ethics. NIX United.
Oxford University Press. (2023, December 30). Ethical Issues. Oxford University Press.
The Hospitalist. (2024, May 1). Ethical AI. The Hospitalist.
The World Economic Forum (2024, September, 25). How AI is improving diagnostics and health outcomes. The World Economic Forum.
About Larrie Hamilton, BHC, MHC
As a medical scientist, I combine research expertise with a passion for clear communication at Biolife Health Center. I investigate innovative methods to improve human health, conducting clinical studies and translating complex findings into insightful reports and publications. My work spans private companies and the public sector, including BioLife and its subsidiaries, ensuring discoveries have a broad impact. I am dedicated to advancing medical knowledge and creating a healthier future. Follow me on LinkedIn.