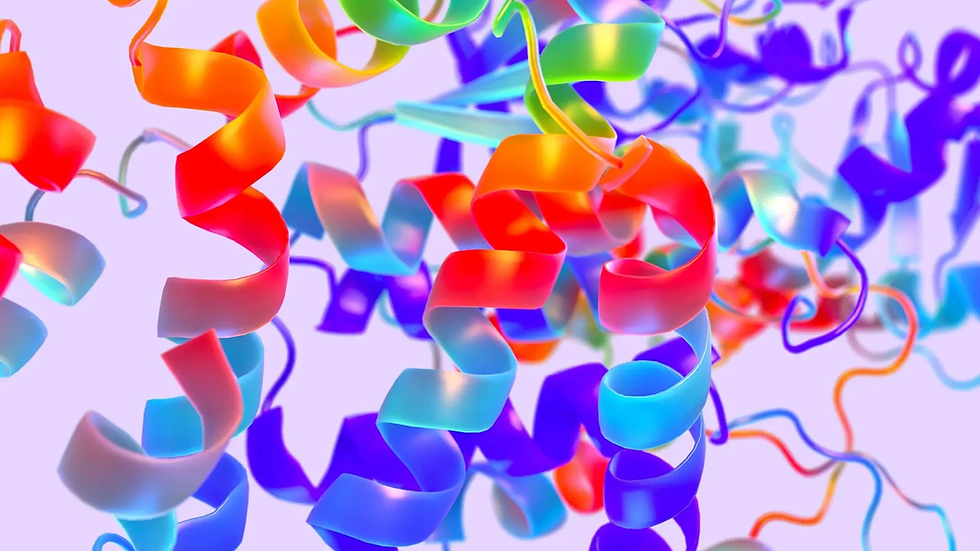
![]() | ![]() | ![]() |
Introduction
It is well known that proteins are considered the fundamental units of the biological system, influencing various functions dependent on their three-dimensional structures. DeepMind's AlphaFold has revolutionized protein structure prediction, driven by advancements in computational biology and Artificial Intelligence (AI). It simultaneously addresses the limitations of conventional methods, which are considered time-consuming and costly.
With AlphaFold’s ongoing advancements, high accuracy, and confidence metrics, the opportunities for discovering known and novel protein structures have expanded to include numerous scientific fields, such as medicine, drug discovery, bioengineering, and environmental sciences. Immerse yourself in the realm of AlphaFold and join us as we explore the challenges faced in protein structure prediction, how AlphaFold addressed these issues, and the significance of this platform for future scientific progress.
Key Component Summary
AI-Driven Revolution: AlphaFold’s mechanism is driven by AI and computational advancements, surpassing traditional methods for accurately and efficiently predicting protein structures.
Overcoming Limitations: It solves the challenges of conventional methods that are time-consuming, costly, and limited by the intricacies of specific protein structures.
Continuous Progression: AlphaFold's different versions, from conception to the currently available model, continuously improve their capabilities, accuracies, and reliability in protein folding prediction.
Machine Learning Innovations: Sophisticated neural networks and modules like Pairformer drive its performance.
Transformative Impact: AlphaFold accelerates scientific advancements in drug discovery, medicine, and bioengineering, with AlphaProteo expanding protein design possibilities.
Proteins: The Driving Force of Biological Systems
Proteins are essential macromolecules that dictate the course of life. They organize activities in the body, form and repair tissues, and carry out all sorts of necessary metabolic processes. They are compounds composed of linear amino acid sequences configured into varying 3-dimensional shapes that determine the protein’s exact cellular function (Sanvictores & Farci, 2022). Because they execute such key processes in the body, proteins have critical applications in understanding disease mechanisms, bioengineering, vaccine development, and many other areas of interest. Thus, elucidating the protein structure profoundly impacts these various scientific fields.
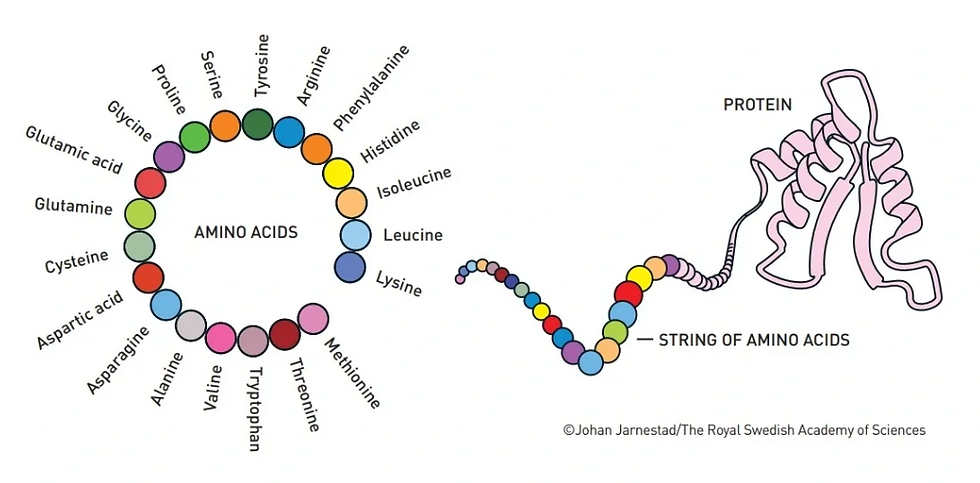
Coined by the Swedish chemist Jacob Berzelius, proteins contain nitrogen and are created via a combination of amino acids formed together by peptide bonds (Fruton, 1985; Wisniak, 2000; Lehninger et al., 2005; Voet et al., 2014; Bertoline et al., 2023). A protein’s function depends on its folding, or structure, consisting of four types: primary, secondary, tertiary, and quaternary. However, proteins can only function at the tertiary or quaternary phases of protein folding, although not all proteins have a quaternary phase (Sanvictores & Farci, 2022). Some of their specific functions include, but are not limited to, cell membrane support, immune system response, enzymatic catalysis, and DNA replication (Sanvictores & Farci, 2022).
Protein Structure Prediction: Traditional Methods Meet AI Models
Given the critical applications in medical research of protein folding predictions, attempts have been made to improve this process, advancing the understanding of disease mechanisms, evolution, and drug interactions. For example, techniques like homology modeling, threading (folding), ab initio methods, X-ray crystallography, cryo-electron microscopy (cryo-EM), and nuclear magnetic resonance (NMR) spectroscopy have previously been attempted; however, these methods have proven too time-consuming, complex and costly (Bertolini et al., 2023; Chen, 2024). Due to these constraints, the number of known tertiary protein sequences (200,988 PDB entries) is significantly lower than the total number of known protein sequences, which, as of November 2024, totals 253,682,368, according to UniProtKB (2024). Furthermore, these methods have struggled to accurately predict the atomic structure of proteins, especially when data is limited, and no homologous protein structures are available for reference (Jumper et al., 2021).
The Challenges of Conventional Methods for Protein Folding
Traditional methods pose different challenges. These include the availability of specialized equipment, experimental procedures that require highly skilled scientists, difficulty predicting complex protein structures due to the protein’s biochemical composition and nature, and predictability or uncertainty based on the prediction outcome, which can only be determined once the experimental component is successful (Qin et al., 2024).
Furthermore, conventional methods also require specific equipment and specialized individuals, which limits the accessibility of the outcomes to this type of science (Qin et al., 2024). In contrast, AlphaFold is an open-source platform accessible to a broad scientific community, where protein information can be obtained through the AlphaFold Protein Structure Database.
Another downside to traditional methods is the limitation of novel protein structure discovery. These methods depend on established and familiar structural templates or similar sequence alignments, challenging the prediction of complex or flexible proteins. This is also the case with dysregulated protein portions, intrinsically disordered protein (IDP) regions with no fixed structures, and the establishment of predictions due to complex linked protein structures (Chen, 2024; Qin et al., 2024). Therefore, the need for faster and even more accurate methods was sought after (Gadde et al., 2024).
Protein Folding Predictions with AlphaFold
With the introduction of AlphaFold, its ability to achieve near-experimental accuracy can be compared to conventional methods. Although AlphaFold's complex protein predictions have advanced structural biology, they require additional validation (Gadde et al., 2024; Kosinki, 2024). Nevertheless, AI can potentially transform this study area by leveraging extensive data storage systems, enhancing pattern recognition, and predicting protein sequences. Overall, this provides a less time-consuming and more cost-effective solution, which can drive advancements in medicine and drug discovery due to its ability to predict multiple structures simultaneously – an impractical approach compared to conventional methods.
Lastly, AlphaFold integrates confidence scores into its software with the predicted local distance difference test (pLDDT), allowing researchers to assess the accuracy and reliability of protein predictions and determine if further validation is needed. It also offers guidance on how AlphaFold can continually refine its protein prediction (Gadde et al., 2024; Wang et al., 2024).
The Evolution of AlphaFold and Its Impact on Protein Structure Prediction
AlphaFold has evolved significantly since its initial development by DeepMind in 2018. It has progressed through multiple iterations, including AlphaFold 2, AlphaFold-Multimer, AlphaFold 3, and the recent AlphaProteo system. Each version has brought substantial advancements to protein structure prediction and design capabilities, revolutionizing our understanding of protein interactions at the molecular level.
AlphaFold 1: The Origin and The First Breakthrough
AlphaFold is a cutting-edge AI algorithm system developed by DeepMind, an offshoot of Google, in 2018 to predict protein structures (The Guardian, 2018). It has undergone numerous versions and iterations since its inception, with each subsequent model improving on the last. The first algorithm model was presented in the thirteenth edition of the Critical Assessment of Structure Prediction competition, or CASP13. CASP is a biennial competition to determine which technological methods can accurately predict protein structure the most (Jumper et al., 2021).
That first AlphaFold model introduced a deep learning system that could predict protein structures more accurately than any other competing system at the time without using any template-based modeling (TBM) (Senior et al., 2019). Its performance was comparable to the best template-based models despite not relying on TBM, marking a groundbreaking achievement in the field (Senior et al., 2019). AlphaFold employs three neural networks: one to predict the distance between pairs of residues in a protein, another to estimate the accuracy of a predicted structure, and a third to generate protein structures (Senior et al., 2019).
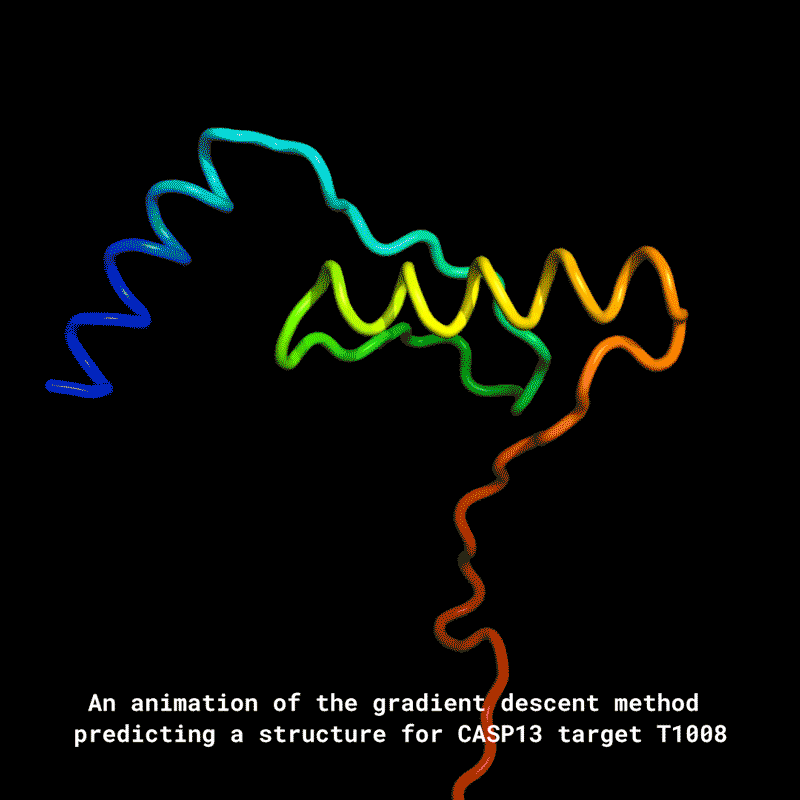
AlphaFold 2: A Revolutionary Leap
Between May and July 2020, during CASP14, a successor model to the first AlphaFold algorithm was introduced, AlphaFold 2, which won the competition (Bertoline et al., 2023). AlphaFold 2 corrected some of the first model's flaws by improving prediction accuracy. First, it introduced revolutionary neural network architectures, such as Evoformer and the structure module (Jumper et al., 2021; Oxford Protein Informatics Group, 2021; Skolnick et al., 2021; Bertoline et al., 2023). The newer model worked by inputting an amino acid sequence to construct a multiple sequence alignment (MSA) to determine which parts of the sequence chain are susceptible to mutations, thus observing the relationship between sequences of different species (Bertoline et al., 2023).
According to the EMBL’s European Bioinformatics Institute (n.d.), an MSA is the alignment of three or more protein or nucleic acid sequences to determine evolutionary similarities and differences in their amino acids. Evoformer works by exchanging information between MSAs and template proteins in a back-and-forth mechanism that improves MSA analysis and refines and adjusts the protein predictions made by the template proteins (Bertoline et al., 2023). The template protein is aligned with other proteins to determine homology or similarity between both sequences in pair representation (Bertoline et al., 2023).
Expanding Understanding of Protein Complexes with AlphaFold-Multimer
Then, in October 2021, AlphaFold-Multimer was introduced as a separate chain of AlphaFold 2 (Evans et al., 2022, as cited in Bertoline et al., 2023). Unlike its predecessor, which only predicted single-chain sequences, AlphaFold-Multimer was designed to predict protein complexes called multimers (Gutnik et al., 2023). AlphaFold-Multimer, like AlphaFold 2, is open-source and readily accessible online (Bertoline et al., 2023). However, it came with its own set of limitations. It has been observed by Jumper et al. (2021) that AlphaFold-Multimer is better at predicting homomeric protein complexes as opposed to heteromeric structures, thus limiting its scope and that it is poor at predicting antigen-antibody binding accuracy (Yin et al., 2022, as cited in Bertoline et al., 2023).
AlphaFold-Multimer’s limitations derive from its dependence on signals found in MSAs for protein interactions. In cases where proteins do not develop together or share coevolutionary patterns, they exhibit weak or no signals. This is seen with heteromeric structures. It is also notable for antigen-antibody binding, where antigens and antibodies do not evolve together in a traditional sense. In essence, it lacks the signals required in MSAs (Evans et al., 2021). In addition, accurate interpretation of binding interfaces in more extensive and intricate structures poses another challenge, leading to low prediction confidence. The flexibility of binding sites associated with antigen-antibodies further complicates predictions, especially for epitopes on the antigenic surface. Furthermore, predictions for structure changes and the quality of MSAs and other input data can cause inconsistent results (Evans et al., 2021).
AlphaFold 3: Enhanced Predictions for Intricate Interactions
However, in May 2024, CASP15 revealed another addition called AlphaFold 3, which augmented the features of its predecessors, AlphaFold 2 and AlphaFold-Multimer v2.3 (Abramson et al., 2024). This latest model revolutionized protein prediction by improving the prediction accuracy of joint structures of various complexes, such as proteins, nucleic acids, ions, and small molecules (Abramson et al., 2024). Unlike AlphaFold-Multimer v2.3, which we mentioned could not predict antigen-antibody binding very well, AlphaFold 3 is adept at predicting the interactions between antigens and antibodies to a higher level of accuracy (Abramson et al., 2024).
Additionally, it has demonstrated better efficiency and accuracy in predicting protein-ligand and protein-nucleic acid sequences than other tools (Abramson et al., 2024). In contrast to AlphaFold 2, AlphaFold 3 reduced the amount of MSA processing by replacing Evoformer with a more straightforward Pairformer module. Furthermore, AlphaFold 3 uses a diffusion module, which, unlike AlphaFold 2’s structure module, can directly pinpoint and predict raw atom coordinates (Abramson et al., 2024).
Protein Design Advancement with AlphaProteo
The most recent innovation from DeepMind, called AlphaProteo, was introduced in September 2024. Instead of predicting proteins based on their amino acid sequence, this AI system generates proteins to bind to specific target molecules (Google DeepMind, 2024). With a myriad of applications, from drug development to cell and tissue imaging to crop resistance, AlphaProteo revolutionizes the medical field by optimizing our understanding of protein interactions at the molecular level (Google DeepMind, 2024). It utilizes data from the Protein Data Bank (PDB) and 100 million predicted proteins from AlphaFold to generate its binding protein given a target protein’s structure and binding sites. The method of generating binding proteins was traditionally exhaustive and time-consuming prior to the advent of AlphaProteo.
AlphaProteo: The Impact on Autoimmune Conditions and More
Although AlphaFold can bind to many target proteins, such as those responsible for infection and cancer, many obstacles remain to overcome with more challenging targets, such as the TNF-α protein. This protein is associated with autoimmune diseases like rheumatoid arthritis, psoriasis, and inflammatory bowel disease (Caldito, 2023; Google DeepMind, 2024). The impact of AlphaProteo on clinical and research applications for autoimmune conditions related to TNF-α is significant. It can contribute to early diagnosis by identifying and validating new biomarkers associated with this protein’s activity (Song et al., 2024). It also enables innovative tools for disease monitoring and treatment efficacy by measuring TNF-α levels (Caldito, 2023). Additionally, AlphaProteo provides insights into the intricate signaling pathways and receptor interactions involving TNF-α, potentially leading to the discovery of new therapeutic targets and enhancing the drug discovery process (Caldito, 2023; Song et al., 2024).
Understanding AlphaFold: What It Does and How It Works
Protein structure prediction has traditionally relied on methods that were considered adequate at the time. However, recent technological advancements are starting to surpass conventional techniques. This is due to the rise of AI technologies and the progression of computational biology as the preferred method for ensuring accuracy and reliability while reducing time constraints and related costs (Guo et al., 2024).
The following section provides an overview of the mechanisms of AlphaFold in an easily understandable way:
Proteins are integral to the human body and are responsible for several health functions. To understand how proteins perform these roles, we must examine their three-dimensional shape. Consider AlphaFold as a very clever AI detective capable of rapidly and accurately identifying the three-dimensional shape of proteins. It uses large amounts of biological data in combination with different methods for this process.
The AI detective starts by gathering clues, using deep learning (DL) to obtain information about protein structures, the connections between protein building blocks, and the rules of protein folding discovered in the past. DL is a type of machine learning that mimics how the brain functions, recognizing specific aspects and relationships between them. The DL strategies consist of convolutional neural networks (CNNs), which can identify patterns, such as how we identify patterns with our eyes. Graph neural networks notice the “social network” of proteins, where amino acids are individuals, and the relationships between them are the connections. The last approach is multiple layer perceptrons (MLPs), which combine data sections to provide a simple and effective prediction result.
Understanding how protein structures are formed requires insight into the order of amino acids that make up the protein. This order is like a code – also known as MSAs – that provides a template for the protein’s shape. The AI detective reviews the arrangement of amino acids based on the different learning strategies to make a prediction. To make this learning and prediction process more manageable, the AI detective uses existing “blueprints” from previous structural templates of proteins, strengthening the outcome of predictions. It can also double-check whether these predictions are accurate.
The heart of the AI detective is Pairformer. It works by integrating the different learning methods and previous blueprints to suggest the best way for the amino acid building blocks to connect and interact, providing the most accurate image. To ensure that the generated picture is accurately predicted, the AI detective uses a confidence score that others can refer to as a measure of reliability. Having the ability to say that a confidence score is high is like a detective stating that he is 99% certain that he caught the correct suspect. This also, in turn, allows his colleagues to focus on the most reliable sections for further investigation, which saves time and resources.
AlphaFold and Computational Biology: Understanding the Intricacies of AI Models
AlphaFold revolutionizes protein structure prediction by employing advanced deep learning to transform amino acid sequences into accurate 3D models. Its core Pairformer component analyzes multiple sequence alignments and structural templates, while neural networks process biological data and evaluate amino acid interactions. The system establishes confidence scores for prediction reliability and employs continuous learning techniques, providing researchers with unprecedented insights into previously challenging protein structures.
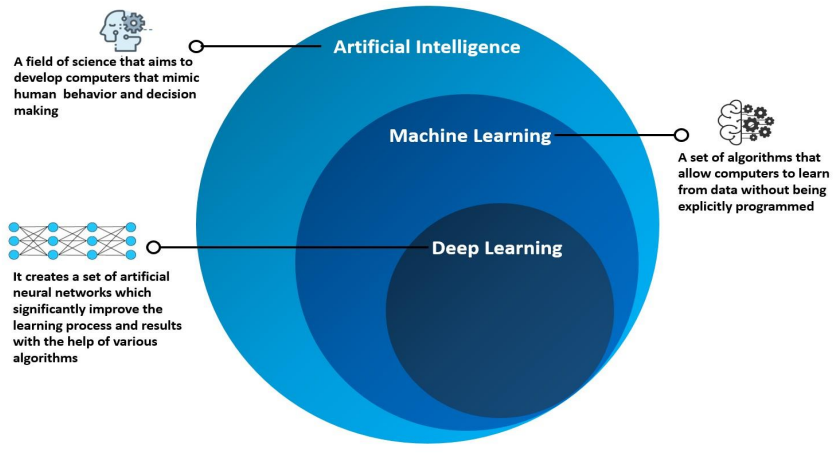
The Source of Protein Prediction Information
Amino acids, the foundation of protein structures, are the primary information that AlphaFold’s neural networks code to provide the template for the protein structure during the prediction process. This input data is transcribed into numerical data and processed by DL architecture (Gadde et al., 2024). The DL mechanism uses CNNs to process consecutive data points, such as amino acid information. This is essential in establishing and understanding the link between amino acid sequences in protein structures (Jumper et al., 2021; Qin et al., 2024).
AlphaFold’s Key Component
Pairformer is the core component of AlphaFold, ensuring accurate predictions based on various integrated factors. Consider it a smart guide that provides instructions on the best way to assemble puzzle pieces. In essence, it combines patterns from earlier versions of a building model and suggests how the building blocks interact and align to create the most accurate picture (Jumper et al., 2021; Qin et al., 2024).
The significance of Pairformer in protein prediction is underscored by its capability to analyze multiple sequences of amino acids (known as MSAs) and structural templates. Think of MSAs and structural templates as a map for exploration. The Pairformer “smart guide” examines this map, reviewing how proteins interact while searching for patterns, highlighting key “landmarks,” and taking precautions before selecting a route. As it reviews the map, it enhances its understanding of amino acid interactions, ensuring the most accurate route by adapting what it has learned, much like a GPS. It navigates between the map and the focal point to consistently identify the best path as the journey unfolds. These processes are essential for providing accurate guidance in protein prediction. This accuracy is further refined by incorporating additional information based on previous data reflecting the evolutionary changes proteins have undergone over time (Jumper et al., 2021; Qin et al., 2024).
Different Data Sources
Historical or evolutionary data comprises the accumulation of previous amino acid sequence versions or protein templates used by Pairformer to identify similarities across different protein sources. Conserved regions or known areas of proteins enhance protein prediction based on similar structural features. This data also highlights inter-relationships between sequences (Jumper et al., 2021; Qin et al., 2024).
An additional source of data includes incorporating external templates to reinforce guidance and predictions from existing databases, such as the PDB (Burley & Berman, 2021; Tejero et al., 2022). The large datasets used for AlphaFold's end-to-end training provide a generalized protein structure prediction for various protein structure families, learning directly from known protein structure samples (Guo et al., 2024).
Another component, MPLs, integrates and refines predictions using combined data from CNNs and Pairformer. MLPs are used because they can process non-linear data, which is preferable for the complexities of protein folding. Therefore, it effectively performs this task by considering various layers and representations of data to deliver accurate predictions for the three-dimensional structures of proteins (Qin et al., 2024).
Evaluating Interactions Between Amino Acids
The AlphaFold model has attention mechanisms focusing on essential and relevant information while simultaneously analyzing multiple data sequences. DL models are applied to this specific function, evaluating the most important interactions between amino acids to predict the final protein structure (Jumper et al., 2021; Qin et al., 2024). Cross-attention layers are applied to gauge the interaction between structural templates and MSAs.
CNNs are integral in spatial detection, depicting proteins as graphs and amino acids as connected points or nodes. Graph neural networks represent the interactions between sequential amino acid base pairs through lines called edges. This reflects the spatial relationship between amino acids and how proteins fold. In turn, the model expresses this information to create a visual three-dimensional structure (Jumper et al., 2021; Tejero et al., 2022).
Establishing Confidence Scores
Furthermore, AlphaFold also uses confidence scores to ensure the reliability of protein prediction. Confidence estimations are developed using statistical and machine learning techniques to assess the protein prediction certainty and compare it to training data variability (Qin et al., 2024). The impact of the confidence scores emphasizes the reliability of protein predictions, with higher confidence scores indicating a greater accuracy (Terwilliger et al., 2023). This enables researchers to focus on high-confidence protein sections for further analysis, driving the investigation for experimental design and hypothesis formulation while contributing to faster research approaches.
However, in cases with limited experimental data, it can be combined as a complementary technique (Terwilliger et al., 2023). Low-confidence structures have been validated through experiments, highlighting AlphaFold’s ability to identify disordered proteins. Moreover, researchers use these confidence scores specifically for proteins that have been difficult to predict in the past, enabling the focus on unexplored areas in protein research (Terwilliger et al., 2023).
Continuous Learning Approaches
Additional protein prediction capabilities include reinforcement learning techniques, whereby protein folding and structural outcomes are simulated to refine the prediction further. This technique also allows the model to adjust its deep-learning architecture as it learns and provides feedback for corrections (Qin et al., 2024). The learning model makes further corrections using gradient flow improvement techniques, such as residual connections, to prevent problems with vanishing gradients or data and information loss. This allows for improved training of deep learning models.
The various aspects of AlphaFold’s architecture enable it to process intricate and vast amounts of biological data, ranging from DL models and neural networks to reinforcement learning, while incorporating known protein templates from large databases. AlphaFold’s dynamic design allows it to produce accurate protein folding predictions, confidently evaluate the predictive structures, contribute insights to computational biology, and clarify the practical application of protein folding in related fields (Wang et al., 2024; Wee & Wei, 2024).
Current Limitations and Ongoing Advancements in AlphaFold
AlphaFold faces key limitations in protein structure prediction, struggling with dynamic structures, non-protein biomolecules, intrinsically disordered regions, and conformational changes. Computational constraints affect accuracy with large or complex proteins, while limited training data and static modeling further reduce effectiveness. Despite these challenges, ongoing improvements through cross-distillation techniques, experimental data integration, enhanced confidence metrics, and complementary tools like ColabFold continue to advance AlphaFold's capabilities.
Limitations influencing AlphaFold’s capability
Due to proteins’ dynamic structure, the challenging aspect of AlphaFold is that it primarily has an advantage in predicting fixed structures, especially with bound and unbound sections of proteins (Abrahamson et al., 2024). Non-protein biomolecules such as DNA and RNA challenge predicting protein interactions with other biomolecules. Furthermore, intrinsically disordered regions (IDRs) that lack stability in the structure are predicted with low confidence (Terwilliger et al., 2023). This leads to potential incorrect structure prediction due to the uncertainty of these unstable regions, such as those with flexible loops or IDRs. Similarly, mutations or shape changes due to binding activities are complex for AlphaFold to predict accurately. Computational challenges also exist where extremely large or intricate proteins and those with long sequences are difficult to predict with high accuracy. At the same time, it also causes a decreased efficiency for the time and resources required for these types of predictions (Abrahamson et al., 2024).
The main difficulties associated with accurately predicting three-dimensional protein structures depend on the availability of training data, such as MSAs and structural databases. Suppose protein structures do not have evolutionary data or new sequences. In that case, this influences AlphaFold’s predictive ability, while the AI mechanisms rely on static proteins and do not account for changing environments (Terwilliger et al., 2023). Where limited data is available, or difficulties exist predicting unstable protein regions, the neural network can “hallucinate” these sections of proteins. Furthermore, the scalability becomes limited for broader applications because of the extensive computational power required for significant predictions (Abrahamson et al., 2024).
The Continuous Progress of AlphaFold
Innovative methods to continually advance AlphaFold are crucial to the scientific community. Incorporating cross-distillation techniques reduces hallucinatory predictions and establishes training techniques on realistic data, including disordered regions or less-explored protein sections. Integrating experimental data into the training dataset, alongside additional AI models and other omics data, improves prediction accuracy, such as through collaboration with AF_unmasked (ScienceDaily, 2024).
Integrating improved confidence metrics, such as pairwise distance error (PDE), aids in prediction reliability at a molecular level and prevents inaccurate predictions.
AlphaFold’s capability is further improved by including post-translational changes and other residues relevant to biological systems in its training data. This provides a broader predictive scope.
Introducing other computational tools, like ColabFold, optimizes resource allocation by exchanging MSAs with more rapid algorithms, such as MMseq2, for faster predictions while maintaining high-quality accuracy (Yin & Pierce, 2024).
The Impact and Recognition of AlphaFold in the Scientific Community
Suppose we had to retrospectively review AlphaFold's impact on the scientific community since its inception. Who would have guessed that in October 2024, DeepMind researchers Demis Hassabis and John Jumper would be awarded the Nobel Prize in Chemistry for their groundbreaking work?
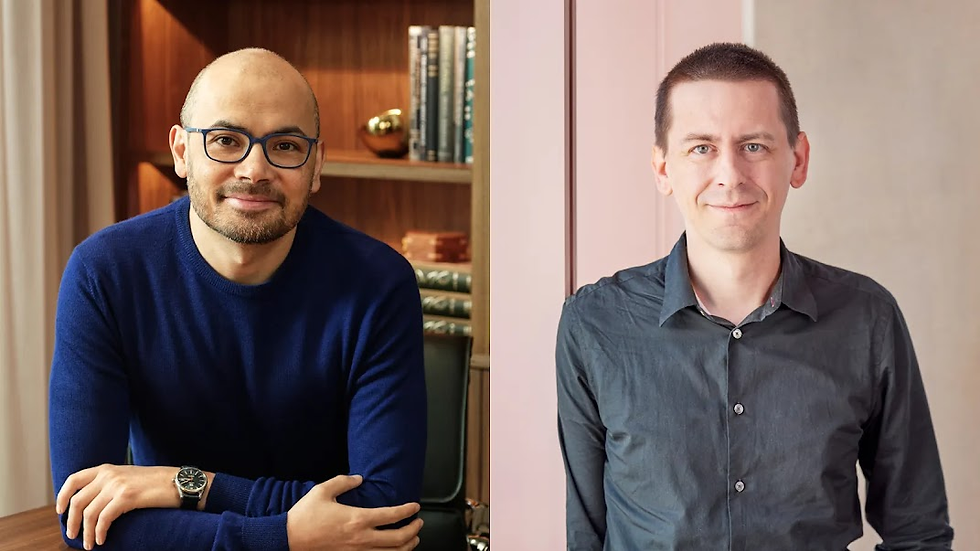
AlphaFold solved the decades-old protein folding problem—predicting a protein's three-dimensional structure from its amino acid sequence—a challenge that had eluded scientists for over 50 years. By employing advanced machine learning techniques, AlphaFold achieved near-experimental accuracy, enabling researchers to determine protein structures in minutes rather than years. This breakthrough has profoundly impacted the scientific community, fundamentally changing how researchers approach molecular biology (Nobel Media AB, 2024).
The influence of AlphaFold extends across numerous disciplines, from drug discovery and enzyme design to the study of complex diseases and agricultural innovation. Its ability to predict protein structures with high accuracy has accelerated research timelines, reducing the need for costly and time-consuming experimental methods. As of 2025, AlphaFold has been cited over 25,000 times and is used by more than two million researchers worldwide, making it one of the most impactful tools in modern science. The system has also democratized access to structural biology, enabling scientists in resource-limited settings to conduct cutting-edge research.
The Nobel Committee emphasized AlphaFold's role in bridging AI and biological sciences, calling it a "transformative tool that has reshaped our understanding of biology." The recognition of Hassabis and Jumper highlights the growing integration between computational methods and experimental science, marking a pivotal moment in scientific innovation. AlphaFold's success has advanced our knowledge of proteins and inspired new approaches to solving other complex scientific challenges, solidifying its legacy as a cornerstone of 21st-century science.
Conclusion
Addressing the limitations of conventional methods, AlphaFold’s AI-driven system simplified protein sequence data and ensured the accurate and efficient prediction of protein structures. It represents a turning point in accelerating structural biology discovery and integrating computational biology while simultaneously providing this essential information to the world through an easily accessible database.
The following articles will continue exploring AlphaFold’s wide range of applications and the endless possibilities it presents through integration with various scientific fields. AlphaFold's continuous advancement provides insights into disease mechanisms, normal biological functions, and how its application further enhances therapeutic drug discovery and impacts environmental science challenges.
Its implications for the scientific world are far-reaching and transformative. They highlight a new era in scientific exploration and shape the innovative approaches and solutions we are moving toward in science.
References:
1. Abramson, J., Adler, J., Dunger, J., Evans, R., Green, T., Pritzel, A., Ronneberger, O., Willmore, L., Ballard, A. J., Bambrick, J., Bodenstein, S. W., Evans, D. A., Hung, C., O’Neill, M., Reiman, D., Tunyasuvunakool, K., Wu, Z., Žemgulytė, A., Arvaniti, E., . . . Jumper, J. M. (2024). Accurate structure prediction of biomolecular interactions with AlphaFold 3. Nature, 630(8016), 493–500. https://doi.org/10.1038/s41586-024-07487-w
2. AI tool AlphaFold can now predict very large proteins. (2024, November 24). ScienceDaily. https://www.sciencedaily.com/releases/2024/11/241104112352.htm
3. Bertoline, L. M. F., Lima, A. N., Krieger, J. E., & Teixeira, S. K. (2023, February 17). Before and after AlphaFold2: An overview of protein structure prediction. Frontiers. https://www.frontiersin.org/journals/bioinformatics/articles/10.3389/fbinf.2023.1120370/full#B42
4. Burley, S. K., & Berman, H. M. (2021). Open-access data: A cornerstone for artificial intelligence approaches to protein structure prediction. Structure (London, England : 1993), 29(6), 515–520. https://doi.org/10.1016/j.str.2021.04.010
5. Caldito, N. G. (2023). Role of tumor necrosis factor-alpha in the central nervous system: a focus on autoimmune disorders. Frontiers in Immunology, 14. https://doi.org/10.3389/fimmu.2023.1213448
6. ChatGPT. (2025). A conceptual illustration of AlphaFold AI receiving a Nobel Prize for its achievement [AI-generated image]. OpenAI.
7. ChatGPT. (2025). A simple doodle-style illustration to aid in understanding protein structures and amino acids [AI-generated image]. OpenAI.
8. ChatGPT. (2025). A simplified infographic illustrating protein structure prediction and AlphaFold [AI-generated image]. OpenAI.
9. ChatGPT. (2025). A straightforward flowchart illustrating the relationship between Machine Learning, Deep Learning, and Convolutional Neural Networks (CNNs) [AI-generated image]. OpenAI.
10. ChatGPT. (2025). A visualization of AlphaFold AI constructing a protein structure [AI-generated image]. OpenAI.
11. Chen, Y. (2024). Advancements and Applications of Protein Structure Prediction Algorithms. Theoretical and Natural Science, 74, 119-127. https://doi.org/10.54254/2753-8818/2024.LA18791
12. EMBL-Ebi Homepage. (n.d.). https://www.ebi.ac.uk/jdispatcher/msa
13. Embl-Ebi. (n.d.). Current release statistics. EMBL-EBI homepage. https://www.ebi.ac.uk/uniprot/TrEMBLstats
14. Evans, R., O’Neill, M., Pritzel, A., Antropova, N., Senior, A., Green, T., Žídek, A., Bates, R., Blackwell, S., Yim, J., Ronneberger, O., Bodenstein, S., Zielinski, M., Bridgland, A., Potapenko, A., Cowie, A., Tunyasuvunakool, K., Jain, R., Clancy, E., . . . Hassabis, D. (2021). Protein complex prediction with AlphaFold-Multimer. bioRxiv (Cold Spring Harbor Laboratory). https://doi.org/10.1101/2021.10.04.463034
15. Gadde, N., Dodamani, S., Altaf, R., & Kumar, S. (2024). Leveraging AlphaFold 3 for Structural Modeling of Neurological Disorder-Associated Proteins: A Pathway to Precision Medicine. bioRxiv.
16. Guardian News and Media. (2018). Google’s DeepMind predicts 3D shapes of proteins. The Guardian. https://www.theguardian.com/science/2018/dec/02/google-deepminds-ai-program-alphafold-predicts-3d-shapes-of-proteins
17. Guo, S. B., Meng, Y., Lin, L., Zhou, Z. Z., Li, H. L., Tian, X. P., & Huang, W. J. (2024). Artificial intelligence alphafold model for molecular biology and drug discovery: a machine-learning-driven informatics investigation. Molecular cancer, 23(1), 223. https://doi.org/10.1186/s12943-024-02140-6
18. Gutnik, D., Evseev, P., Miroshnikov, K., & Shneider, M. (2023). Using alphafold predictions in viral research. Current issues in molecular biology. https://pmc.ncbi.nlm.nih.gov/articles/PMC10136805/#:~:text=AlphaFold%2DMultimer%20was%20designed%20to,of%20cases%20of%20homomeric%20interfaces.
19. Jumper, J., Evans, R., Pritzel, A., Green, T., Figurnov, M., Ronneberger, O., Tunyasuvunakool, K., Bates, R., Žídek, A., Potapenko, A., Bridgland, A., Meyer, C., Kohl, S. A. A., Ballard, A. J., Cowie, A., Romera-Paredes, B., Nikolov, S., Jain, R., Adler, J., Back, T., … Hassabis, D. (2021). Highly accurate protein structure prediction with AlphaFold. Nature, 596(7873), 583–589. https://doi.org/10.1038/s41586-021-03819-2
20. Kosinski, J. (2024). Improving AlphaFold 3 structural modeling by incorporating explicit crosslinks. bioRxiv.
21. Nobel Media AB. (2024). The Nobel Prize in Chemistry 2024. https://www.nobelprize.org
22. Protein Design and Wet Lab teams. (2024, September 5). Alphaproteo generates novel proteins for biology and Health Research. Google DeepMind. https://deepmind.google/discover/blog/alphaproteo-generates-novel-proteins-for-biology-and-health-research/
23. Qin, Y., Chen, Z., Peng, Y., Xiao, Y., Zhong, T., & Yu, X. (2024). Deep learning methods for protein structure prediction. MedComm – Future Medicine, 3(3). https://doi.org/10.1002/mef2.96
24. Sanvictores, T., & Farci, F. (2022, October 31). Biochemistry, primary protein structure. StatPearls [Internet]. https://www.ncbi.nlm.nih.gov/books/NBK564343/
25. Senior, A. W., Evans, R., Jumper, J., Kirkpatrick, J., Sifre, L., Green, T., Qin, C., Žídek, A., Nelson, A. W. R., Bridgland, A., Penedones, H., Peterson, S., Simonyan, K., Crossan, S., Kohli, P., Jones, D. T., Silver, D., Kavukcuoglu, K., & Hassabis, D. (2019, October 10). Protein structure prediction using multiple deep neural networks in the 13th critical assessment of Protein structure prediction (casp13). https://onlinelibrary.wiley.com/doi/10.1002/prot.25834
26. Song, Y., Li, J., & Wu, Y. (2024). Evolving understanding of autoimmune mechanisms and new therapeutic strategies of autoimmune disorders. Signal Transduction and Targeted Therapy, 9(1). https://doi.org/10.1038/s41392-024-01952-8
27. Tejero, R., Huang, Y. J., Ramelot, T. A., & Montelione, G. T. (2022). AlphaFold Models of Small Proteins Rival the Accuracy of Solution NMR Structures. Frontiers in molecular biosciences, 9, 877000. https://doi.org/10.3389/fmolb.2022.877000
28. Terwilliger, T. C., Liebschner, D., Croll, T. I., Williams, C. J., McCoy, A. J., Poon, B. K., Afonine, P. V., Oeffner, R. D., Richardson, J. S., Read, R. J., & Adams, P. D. (2023). AlphaFold predictions are valuable hypotheses and accelerate but do not replace experimental structure determination. Nature Methods, 21(1), 110–116. https://doi.org/10.1038/s41592-023-02087-4
29. Wang, J., Sun, H., Mou, L., Lu, Y., Wu, Z., Pu, Z., & Yang, M. M. (2024). Unveiling the molecular complexity of proliferative diabetic retinopathy through scRNA-seq, AlphaFold 2, and machine learning. Frontiers in endocrinology, 15, 1382896. https://doi.org/10.3389/fendo.2024.1382896
30. Wee, J., & Wei, G. W. (2024). Evaluation of AlphaFold 3's Protein-Protein Complexes for Predicting Binding Free Energy Changes upon Mutation. Journal of chemical information and modeling, 64(16), 6676–6683. https://doi.org/10.1021/acs.jcim.4c00976
31. Yin, R., & Pierce, B. G. (2024). Evaluation of AlphaFold antibody-antigen modeling with implications for improving predictive accuracy. Protein science : a publication of the Protein Society, 33(1), e4865. https://doi.org/10.1002/pro.4865
Lead Researcher and Writer: Renaldo Pool, BHSc Research and Writing: David Matin, B.A. and Cindy Hamilton, MPH Review & Editing: Larrie Hamilton, MHC Visualization: Michelle Ryan, MHA Conceptualization and Methodology: David Priede. PhD Project Administration and Funding Acquisition: BioLife Health Research Center |